Mitha Rachel Jose
Heikki Kaakinen
Janne Känsäkoski
Blockchain is one the most hyped innovations these days and it has been gaining a lot of popularity as a horizontal technology to be widely adopted in various fields (Maxmen 2018). Artificial Intelligence (AI) plays a substantial role in making analytic tools and delivering a scalable and precise analysis of data in real-time. The primary goal of our study is the integration of Blockchain and AI to support secure, decentralized data analysis for Internet of Things (IoT) applications in industries (Singh, Rathore & Park 2020). This study contributes the integration of artificial intelligence, especially machine learning (for collecting data from industry), and the hash of those data will be shared in Blockchain and the real data will be secured with MyData. Finally, the application of different smart technologies leads to an environment of less carbon emission.
Rui et al. (2016) studied the reduction of CO2 emissions to 41 industrial sectors of China by using a method by combining an input-output analysis and entropy weighting. This was done in two ways in which the first one tries to calculate the carbon emission intensity and the second way was to find the emissions’ reduction weighting of each industry/sector.
Blockchain is a pioneering technology authorizing the benign and reliable storage and transmission of data, among its other advantages. Artificial Intelligence, on the other hand, is an innovative technology that can learn by its own to analyse and determine patterns in enormous amounts of data. Smart contract-based autonomous systems and machines can learn and adapt to changes over time and make trusted and accurate decision outcomes that are verified and validated by all mining nodes of the Blockchain. Such decisions cannot be countered and can be traced, tracked and verified by all participating entities. The aim of this study is,
- a concrete integration of machine learning algorithms with Blockchain leads to better QoS characteristics, reducing power consumption and reduction in overall cost
- reducing the challenge of storing large files in Blockchain thereby reducing latency
- to help in achieving a less carbon emission environment by the first two steps.
The background of this study is based on four main know-hows a) Blockchain b) Artificial Intelligence c) Transformation of Blockchain to Artificial Intelligence and d) MyData.
- Blockchain is a new technology that redefines the way the transactions are happening through the internet. It is a transformation that has already begun. Blockchain has the potential to change the way we buy and sell, interact with government, and verify the authenticity of everything from property to organic vegetables. The organizations and the middlemen at the risk of disintermediation will need to be prepared as technology matures. Blockchain gives everyone a safer and faster way to verify key information and establish trust.
Conventional blockchain is a very expensive medium for storing large amounts of data. For example, storing large files or documents on Bitcoin blockchain is very expensive as the size limit per block is limited to one megabyte (Hartman, Murdock & Spalink 1999). To solve this problem, a decentralized storage medium is used for storing such data and hashes of the data are linked with the blockchain blocks or used within the blockchain smart contract code. Among the popular decentralized storage technologies are the Interplanetary File System (IPFS), Swarm (Benet 2014), Filecoin (Psaras & Dias 2020), BigChainDB (McConaghy et al. 2016), Storj (Wilkinson et al. 2014), and many others.
In order to prevent spamming when instantiating or invoking smart contracts on a block chain, it is often required to pay a fee (Brincat, Lombardo & Morabito 2019), e.g. health care and medical records, identity management, scaling block chains. Permissioned smart contracts are residing on permissioned block chains. The security of a smart contract needs to be built properly in order for it to be considered safe to use. They are more reliable, secure, efficient, and trustworthy as compared with paper contracts. The working of a smart contract depicts in figures 1 and 2.
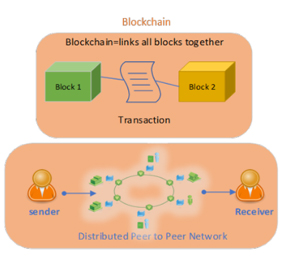
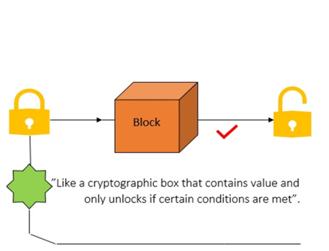
The figure 3 shows the benefits of using blockchain.
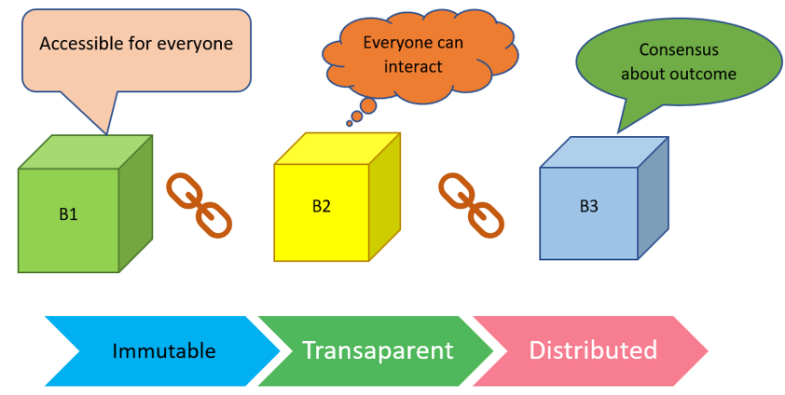
- Artificial Intelligence: Any automatic machine can perform various functions, such as perceiving, learning, reasoning, and the process of solving a problem is automatically known as artificial intelligence.
Machine learning, deep learning, and neural network are used for solving the complex problem automatically, and the benchmark for AI is the human level concerning reasoning, speech, and vision (Singh et al. 2020).
AI takes raw data as input and performs decision-making, and finally gives the maximum outputs for a specific purpose (Rathore et al. 2017).
The figure 4 shows the different ways of artificial intelligence methods and its benefits.
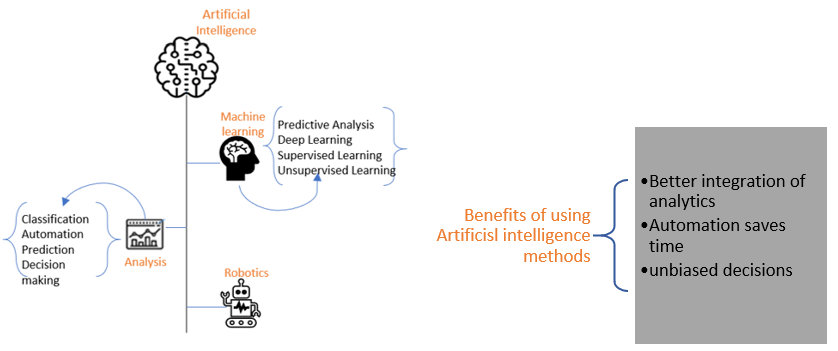
- Transformation of Blockchain to Artificial Intelligence: AI algorithms rely on data or information to learn, infer, and make final decisions. The machine learning algorithms work better when data are collected from a data repository or a platform that is reliable, secure, trusted, and credible. Blockchain data are stored with high integrity and resiliency and cannot be tampered with. When smart contracts are used for machine learning algorithms to make decisions and perform analytics, the outcome of these decisions can be trusted and undisputed. The consolidation of AI and blockchain can create secure, immutable, decentralized system for the highly sensitive information that AI-driven systems must collect, store, and utilize. This concept results in significant improvements securing the data and information in various fields, including medical, personal, banking, and financial, trading, and legal data. (Singh et al. 2020)
- MyData: The term MyData refers 1) to a new approach, a paradigm shift in personal data management and processing that seeks to transform the current organization centric system to a human centric system, 2) to personal data as a resource that the individual can access and control. Personal data that is not under the respective individual’s own control cannot be called MyData. The aim is to provide individuals with the practical means to access, obtain, and use datasets containing their personal information, such as purchasing data, traffic data, telecommunications data, medical records, financial information and data derived from various online services and to encourage organizations holding personal data to give individuals control over this data, extending beyond their minimum legal requirements to do so. (Poikola, Kuikkaniemi & Honko 2015)
Proposed Method: The application of the latest digital methods of improving the energy and material efficiency of selected production processes in large-scale industry is unique at the national and international levels.
A key challenge in developing a low carbon industrial strategy is that the route to a low carbon energy system is typically uncertain. In industry, it is important to identify the economic benefits of ecological operations such as material savings and efficiency, streamlining of processes, the company’s brand, and reputation. These all are financially rewarding parts of a business. So, saving energy through sustainable processes means saving money and even extra profit.
From a low-carbon perspective, it is very illustrative that modifying environment mainly means updating and developing software. Data is gathered from the sensors placed in pilot plants (industries). The collected data which is processed using machine learning algorithms are secured using blockchain technology and stored using MyData. The data are analysed using artificial intelligence (AI). Those data are then accessible from the application programming interface (API) which then can be shown to user through the user interface. The design overview of the proposed BlockIntelligenceData architecture is shown in fig. 5.
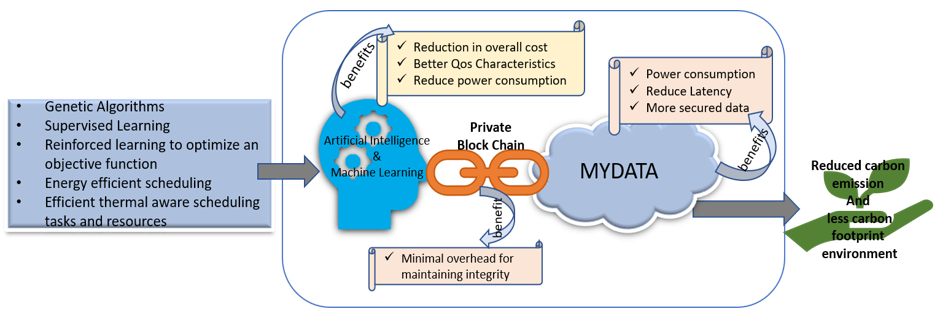
Methods:
Artificial Intelligence: The AI has a wide application connecting the stacks of information inside the system of record or from a silo of database and predicts the next action. This helps to collect, interpret, select, and organize data from different environmental systems using a centralized approach which leads to a huge collection of data. Saleh, Dzakiyullah & Nugroho (2016) proposed an SVM model to predict the expenditure of carbon (CO2) emission. The energy consumption, such as electrical energy and burning coal, is an input variable that affects directly to increasing of CO2 emissions. This information was used to build the model. Besides, the blockchain enables immutable and highly secure versioning of learning models by maintaining the provenance and historical aspects of data. However, considering the permanent nature of smart contracts, learning models need to be trained and verified well prior to deployment on blockchain (BlockApps 2021).
Private Blockchain: The blockchain smart contracts use cases include the use in issuing prescriptions in hospitals, storing receipts, general stock management, storing test results, and so on. Carbon footprint is the total amount of carbon dioxide emitted to the surrounding by companies, individuals, events, or products. An industry solution by “Trace Harvest” describes Energy Usage Tracking and Carbon Credit (CO2) Calculation. This solution explains the measure and calculates the annual report of the carbon impact of the industry, performs cost benefit analysis and automates and monetizes carbon credits (BlockApps 2021). A blockchain enabled carbon footprint solution is demonstrated by a team of blockchain experts at LeewayHertz (2018). In this solution, they described a blockchain enabled carbon footprint process. Here the incoming data are processed by using a smart contract to generate the carbon footprint report of a company, individual or a product. A case study implementation in the Danish energy industry shows a blockchain technology for monitoring and reporting of carbon emission trading. The objective of this study is the conceptual model of blockchain that will be presented and will be limited to only monitoring and reporting of CO2 emission trading for the Danish energy industry (Osuuji 2020).
Machine Learning: Learning plays key role in AI applications to understand the environment from current data and perform informed decision-making based on new data.
Machine Learning methods are used for the reduction of greenhouse gas emissions in the transport & logistics sector. In short, Artificial Intelligence can help large companies to reduce their environmental impact and can improve the efficiency in production, transportation and thereby reducing carbon emissions and cutting costs.
MyData: MyData is an infrastructure-level approach to ensuring data interoperability and portability. The machine learnt large files of data could be stored in a human centric system called MyData (Poikola et al. 2015). The hash values of those data will be stored on the blockchain and the data are managed across the network using token-based incentives for nodes storing different shards or participating in the swarms (Hartman et al. 1999).
The figure 6. explains the flow of data between blockchain, machine learning and MyData.
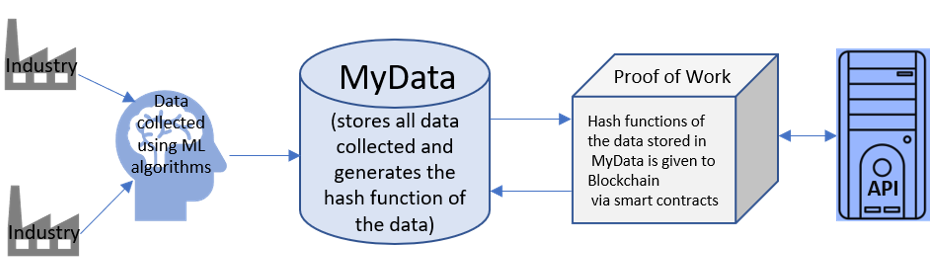
Conclusion
With the integration of smart technologies, such as machine learning, artificial intelligence and blockchain, the carbon emissions can be reduced. This is gained by the analysis and monitoring of energy consumption by machine learning. The goal of the proposed BlockIntelligenceData architecture which tries to converge artificial intelligence, blockchain and MyData may resolve the problem of accuracy, latency, and issues. A decentralized approach is used to get an improved energy consumption, scalability, interoperability, security and privacy, efficiency, resource management, and the integrity of data flows.
References:
BLOCKAPPS, 2021. Energy Usage Tracking and Carbon Credit (CO2) Calculation. BlockApps.
Benet, J. 2014. IPFS – Content Addressed, Versioned, P2P File System. arXiv preprint arXiv:1407.3561.
Brincat, A. A., Lombardo, A., Morabito, G. & Quattropani, S. 2019. On the use of Blockchain technologies in WiFi networks. Computer Networks, 162, pp. 106855.
Hartman, J. H., Murdock, I. & Spalink, T. 1999. The Swarm scalable storage system. 19th IEEE International Conference on Distributed Computing Systems, June 1999, IEEE, pp. 74-81.
Maxmen, A. 2018. AI researchers embrace Bitcoin technology to share medical data. Nature, 555(7696), pp. 293-294.
Mcconaghy, T., Marques, R., Müller, A., De Jonghe, D., Mcconaghy, T., Mcmullen, G., Henderson, R., Bellemare, S. & Granzotto, A. 2016. Bigchaindb: a scalable blockchain database.
Singh, S. K., Rathore, S. & Park, J. H. 2020. BlockIoTIntelligence: A Blockchain-enabled Intelligent IoT Architecture with Artificial Intelligence. Future Generation Computer Systems, 110, pp. 721-743
Osuji, N. 2020. Blockchain Technology for Monitoring and Reporting of Carbon Emission Trading. A case study on its possible implementation in the Danish energy industry. Master Thesis. Technical Faculty of IT and Design. Aalborg University in Copenhagen.
Poikola, A., Kuikkaniemi, K. & Honko, H. 2015. MyData – A Nordic Model for human-centered personal data management and processing. Ministry of Transport and Communications.
Psaras, Y. & Dias, D. 2020. The InterPlanetary File System and the Filecoin Network, 2020, IEEE. 50th Annual IEEE-IFIP International Conference on Dependable Systems and Networks-Supplemental Volume (DSN-S), pp. 80
Rathore, S., Sharma, P. K. & Park, J. H. 2017. XSSClassifier: An Efficient XSS Attack Detection Approach Based on Machine Learning Classifier on SNSs. Journal of Information Processing Systems, 13(4), pp. 1014-1028.
Rui Z., Ning M, Yong G. & Yulong H. 2016. Allocation of carbon emissions among industries/sectors: An emissions intensity reduction constrained approach. Journal of cleaner Production, 142, DOI: 10.1016/j.jclepro.2016.10.159
Saleh, C., Dzakiyullah, N. R. And Nugroho, J. B. 2016. Carbon dioxide emission prediction using support vector machine. IOP Conference Series Materials Science and Engineering 114(1), pp. 012148. DOI: 10.1088/1757-899X/114/1/012148.
The Team of Blockchain Experts at Leewayhertz. 2018. Blockchain Reducing Carbon Footprints – Environmental Impact.
Wilkinson, S., Boshevski, T., Brandoff, J. & Buterin, V. 2014., Storj. A Peer-to-Peer Cloud Storage Network.
Mitha Rachel Jose
RDI-developer
Centria University of Applied Sciences
tel. +358 40 352 5297
Heikki Kaakinen
RDI-expert
Centria University of Applied Sciences
Janne Känsäkoski
Development Manager
Centria University of Applied Sciences

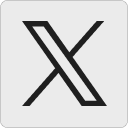

